Angie Laird
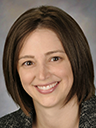
Speaker of Workshop 2
Will talk about: Data-driven approaches for deriving a semantic framework for cognitive paradigms
Dr. Laird is a cognitive neuroscientist whose research program aims to understand the functional organization of large-scale brain networks. Her work focuses on developing novel data analysis algorithms, neuroscience informatics tools, and neuroimaging ontologies to yield analytic strategies for improving investigations into functional brain networks of healthy individuals, as well as in populations with psychiatric and neurologic diseases and disorders. Dr. Laird received her B.S. in Physics from Florida State University in 1998, and her Ph.D. in Physics/Medical Physics from the University of Wisconsin-Madison in 2002. She joined the faculty at the Research Imaging Institute of the University of Texas Health Science Center San Antonio in 2005. In 2010, Dr. Laird was recognized as a “Rising STAR” by the University of Texas System. Her research is currently funded by awards from the National Institutes of Health. In 2012, Dr. Laird moved to Miami and is currently an Associate Professor in the Department of Physics at Florida International University.
A massive amount of functional neuroimaging data is being acquired, analyzed, and published to provide a more complete understanding of the organization and interactions between cortical and subcortical brain regions that enable human cognition. Two decades of progress in neuroinformatics research is now coming to fruition, as neuroimaging databases are being used to aggregate, synthesize, and mine the collective work of the neuroimaging community. In my talk, I will discuss a BrainMap-based approach for knowledge representation in functional neuroimaging data, and will describe the development of a cognitive paradigm ontology. In addition, I will highlight recent findings for developing a novel method for determining paradigm sub-classification in an automated, data-driven fashion, based on the premise that meaningful segregations in tasks should result in observable dissociations in brain activation patterns. Exemplar results will be provided in the context of paradigm classifications during face perception and discrimination.