Michael Wibral
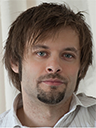
Speaker of Workshop 4
Will talk about: Graphical analyses in delayed interaction networks
Prof. Dr. Michael Wibral is a professor in Magnetoencephalography at the Johann Wolfgang Goethe University of Frankfurt am Main where, since 2006, he is head of the MEG unit / Brain Imaging Center. He received his Bachelor's degree in Physics from the University of Cologne and subsequently earned the Master's degree equivalent from the University of Konstanz. In 2007 he obtained his PhD from the Technical University of Darmstadt after having concluded his PhD studies at the Max Planck Institute for Brain Research in Frankfurt am Main, Department of Neurophysiology headed by Professor Singer. He is also a fellow at the Ernst Strüngmann Institute for Neuroscience in Frankfurt and simultaneously a member of the board and the speaker for the NeFF-LOEWE Initiative. In this capacity, in 2012 he organized the NeFF-Workshop on Non-linear and model-free Interdependence Measures in Neuroscience. Prof. Dr. Michael Wibral main research subject is Transfer Entropy(TE) as a model free measure of effective connectivity in neuroscience and the development of the open source toolbox TRENTOOL.
Network or graph theory has become a popular tool to represent and analyze large-scale interactions in the brain. To derive a network representation from recorded time series we have to identify the structure of the interactions between these time series. This is commonly done by pairwise bivariate analysis as a fully multivariate treatment is often not possible due to limited data. Furthermore, a true multivariate analysis would consist of the analysis of the combined effect of all possible subsets of network components (their power set), leading to a combinatorial explosion (i.e. a problem that is computationally tractable). Pairwise bivariate analysis introduces the possibility of the detection of spurious interactions between network components due to cascade effects and common drive effects. Spurious connections in a network representation may introduce a bias to subsequently computed graph theoretical measures as these measures depend on the reliability of the graph representation from which they are computed (e.g. clustering coefficient or centrality).Strictly speaking, graph theoretical measures are meaningful only, if the underlying graph structure can be guaranteed to consist of one type of connections only, i.e. connections in the graph are guaranteed to be non-spurious. We propose an algorithmic approach to flag potentially spurious edges due to cascade effects and “simple” common drive effects in a network representation of bivariately analyzed interactions. This approach is based on the detection of directed interactions and the weighting of these interactions by their reconstructed interaction delays. We demonstrate how both questions can be addressed using a modified estimator of transfer entropy. Transfer entropy is an implementation of Wiener’s principle of observational causality based on information theory, and detects arbitrary linear and non-linear interactions. Using a modified estimator that uses delayed states of the driving system, we provide a mathematical proof that transfer entropy values peak if the delay of the state of the driving system equals the true interaction delay. From this analysis, we derive a delay weighted network representation of directed interactions. The proposed algorithm may be used to partially prune spurious edges from this network, improving the reliability of the network representation itself and enhancing the applicability of subsequent graph theoretical measures. For the detection of complex common drive effects, a theoretical but no implemented solution exists, yet. This post hoc correction may be a computationally less demanding alternative to a fully multivariate analysis of directed interactions, in cases were a multivariate treatment of the data is not possible.