Iya Khalil
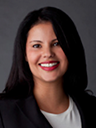
Speaker of Workshop 3
Will talk about: Applying causal inference modeling in multiple sclerosis
Iya Khalil is the executive vice president and co-founder of GNS Healthcare, a big data analytics company that has developed an industrialized analytics platform to extract cause-and-effect relationships directly, and at scale, from observational data. Her goal is to leverage these capabilities to transform medicine from an expert-driven field – with poor predictive power and large gaps in its fundamental understanding of human biology – into a data-driven predictive science with a more complete understanding of the mechanisms of disease and response to interventions. As the co-founder and co-inventor of the company’s proprietary computational platform, she has played a critical role in both technology development and the application of the technology to challenges in medicine, healthcare, and biomedical discovery. Most recently, her focus has been on commercializing the platform in biomedicine and demonstrating its ability to identify predictive biomarkers for drugs in clinical development, treatment algorithms for predicting patient outcomes, and new targets for therapeutic interventions. As a result of these efforts, GNS has forged strong industry partnerships with major pharmaceutical and biotech companies and is on a path to significant commercial growth in the biopharmaceutical space.
We have the opportunity today to harness the power of computer modeling and shared data to create a paradigm shift in our understanding of the mechanisms underlying disease. The work I will describe leverages multiple types of data from patients with multiple sclerosis –genetic, molecular, phenotypic, and clinical – to build models in an unbiased way. Causal disease models developed directly from data provide the predictive power we need to improve clinical outcomes for patients. Knowledge of the essential factors that drive the disease course in individual patients allows us to provide the right treatment at the right time to each patient.
Our approaches to computer modeling include reverse engineering of networks directly from data, followed by in-silico simulations to generate predictions. We also embed clinical validation and iterative improvements in our paradigm in order to continually refine our models.