Rob Kass
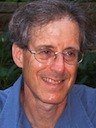
Speaker of Workshop 1
Will talk about: Point process regression models of neural synchrony
Rob Kass is Professor in the Department of Statistics, the Machine Learning Department (School of Computer Science), and the Center for the Neural Basis of Cognition at Carnegie Mellon University. His early research was on differential geometry in statistics and on Bayesian inference. He has been involved in computational neuroscience since 1997, and is interested in all aspects of analysis of neural data. The bulk of his neuroscience publications have concerned the analysis of neural spike trains.
Over roughly the past 10 years point process regression models, often called generalized linear models or GLMs, have become a standard tool for relating neural spiking activity to putative causes, such as features of stimuli, network dynamics, and intrinsic dynamics of neurons. I will begin by discussing the virtues of this modeling approach, and will then turn to the problem of assessing neural synchrony. Synchrony is widely believed to play a fundamental role in neural computation, but its statistical assessment is subtle. I will describe how point process regression models generalize what are commonly called maximum entropy models, and can accommodate the kind of time-varying firing rates that typically appear in stimulus-driven (or action-driven) neurons, as well as non-Poisson or network effects. One of the problems faced in large array recordings is the statistical control of false discoveries. A Bayesian method for controlling false discoveries has yielded interesting physiological results from Utah array recordings in primary visual cortex.