Jonathan Pillow
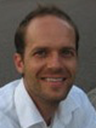
Speaker of Workshop 1
Will talk about: Scalable nonparametric models for large-scale neural datasets
Jonathan received a Ph.D. in neuroscience from New York University in 2005, working with Eero Simoncelli on statistical models of spike trains in the early visual pathway. He completed a postdoctoral fellowship at the Gatsby Computational Neuroscience Unit at University College London, and in 2009 began an assistant professorship at the University of Texas at Austin, with affiliations to the Center for Perceptual Systems, Department of Psychology, Section of Neurobiology, and Division of Statistics and Scientific Computation. Jonathan completed his undergraduate education at the University of Arizona in Tucson, where he studied mathematics and philosophy as a Flinn Scholar. In 1997-98, he studied North African literature as a Fulbright Fellow in Morocco. Jonathan's current research focuses on the neural code, with an emphasis on statistical methods for neural data analysis and probabilistic theories of information processing in the brain.
Statistical models for binary spike responses provide a powerful tool for understanding the statistical dependencies in large-scale neural recordings. Maximum entropy (or "maxent") models, which seek to explain dependencies in terms of low-order interactions between neurons, have enjoyed remarkable success in modeling such patterns, particularly for small groups of neurons. However, these models are computationally intractable for large populations, and low-order maxent models do not accurately describe many datasets. To overcome these limitations, I will describe a family of ``universal'' models for binary spike patterns, where universality refers to the ability to model arbitrary distributions over all possible 2^M binary patterns. The basic approach, which exploits ideas from Bayesian nonparametrics, consists of Dirichlet process combined with a well-behaved parametric "base" model, which naturally combines the flexibility of a histogram and the parsimony of a parametric model. I will explore computational and statistical issues for scaling these models to large-scale recordings and show applications to neural data from primate V1.